DBT London Experts in DBT Therapy
Get Personalised DBT Treatment
Relief for Emotional Dysregulation, BPD, and More
In-Person and Online DBT Therapy Sessions with DBT London
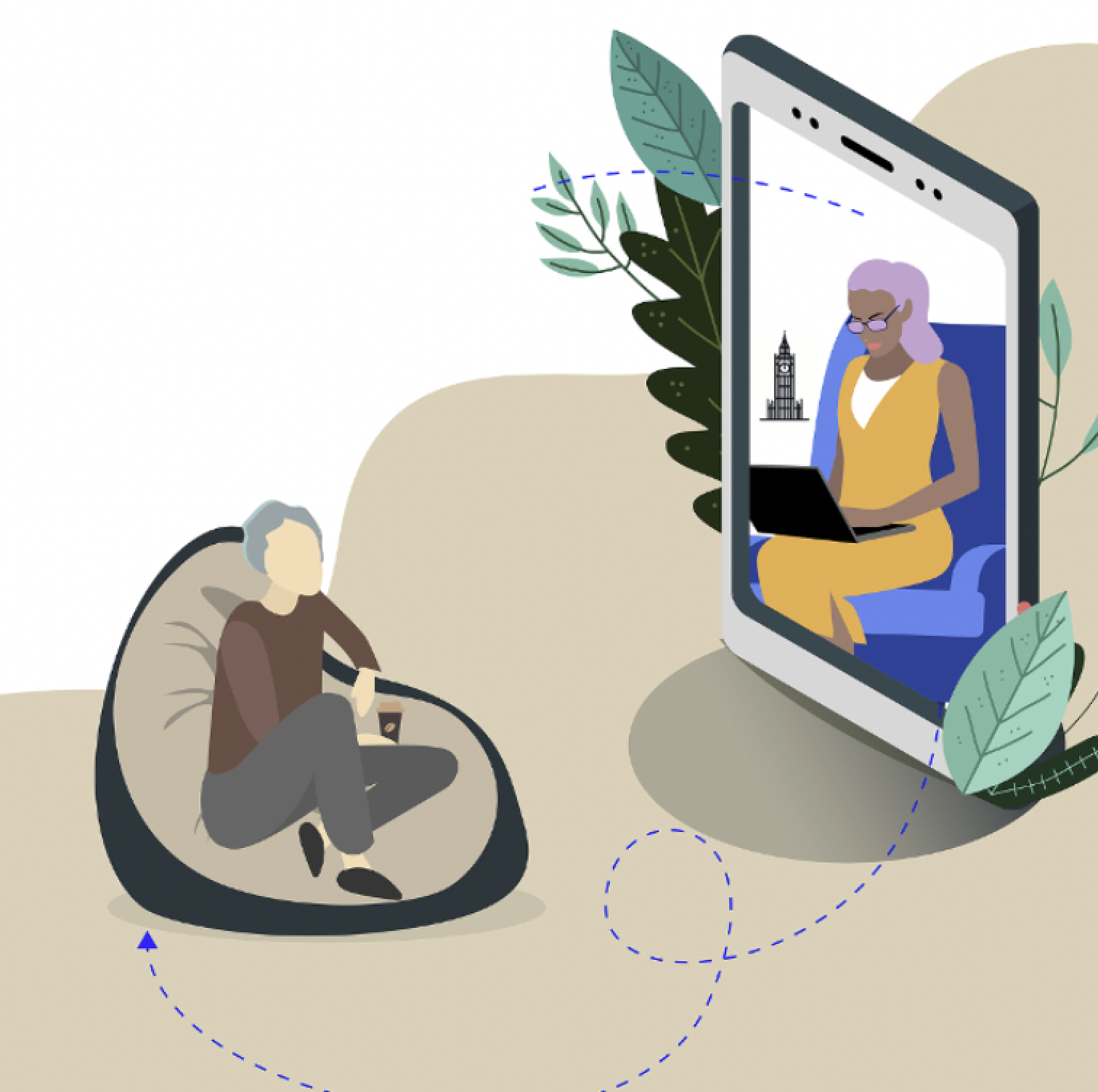
Step 1: Personalised Assessment with DBT London
At DBT London, your path to well-being begins with a detailed, personalised DBT assessment. It’s convenient and friendly, once your payment is confirmed, you’ll receive a Zoom link for a one-on-one session with one of our expert DBT therapists, specialising in DBT Skills for Emotional Regulation and Borderline Personality Disorder (BPD). Together, we’ll determine if DBT online is the right solution for your needs.
Fee: £85 for a one-hour, comprehensive DBT therapy session and treatment plan.
If DBT seems like the right choice, we’ll proceed with the next step a complimentary precommitment session, ensuring a mutual commitment for a successful therapeutic journey.
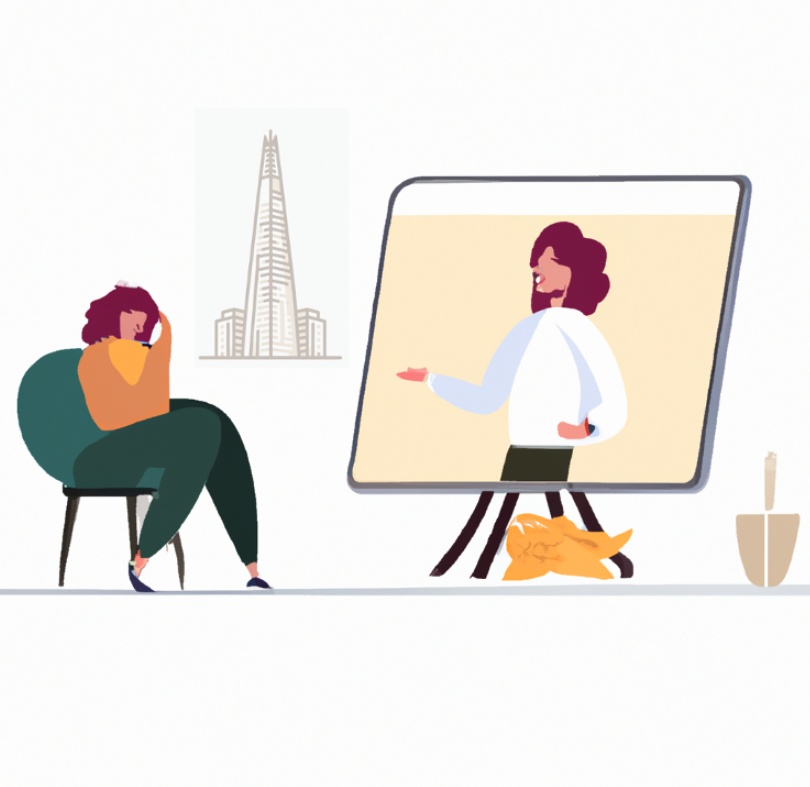
Step 2: Supportive Online DBT Precommitment Session
Before diving into the intensive DBT online therapy, we offer a complimentary precommitment session. This session is your gateway to better understand DBT therapy, establish a trusting bond with your therapist, clarify expectations, and address any concerns or reservations.
Why it’s Needed:
Builds a Foundation and forms a trusting relationship with your DBT therapist.
Assures Mutual Commitment and aligns your commitment with your therapist’s for a successful DBT therapy experience.
Clarifies Expectations and Addresses Concerns, provides insight into the DBT therapy process and addresses concerns right from the start.
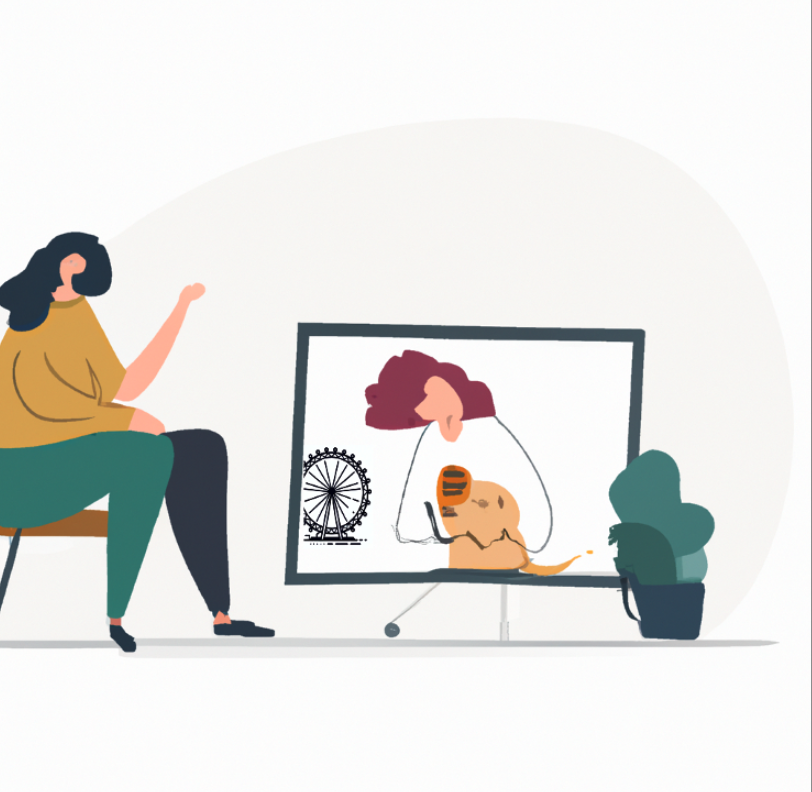
Step 3: Empowering DBT Online Treatment
Begin your 30-week intensive DBT online journey with your dedicated therapist from DBT London. Each session is designed to provide you with practical skills to overcome emotional instability and interpersonal challenges, core components of DBT therapy.
DBT London Programme Offers:
30-Week Intensive DBT Skills Group Programme: Engage in supportive weekly online sessions with each group facilitated by two DBT therapists.
Inclusive supportive weekly online 121 DBT sessions alongside the DBT skills groups with your dedicated DBT Therapist. Your 121 therapist will also provide Crisis Coaching when needed.
Inclusive online monthly Family Support Group: Our continuous support ensures you’re family or partner are a part of your journey.
Once patients have completed the DBT programme and are discharged, they are eligible to join our FREE 10-week remote aftercare group.
Learn DBT Skills with the DBT London YouTube Channel
Expand your knowledge of Dialectical Behavior Therapy through DBT London’s dedicated YouTube channel. Get valuable insights to help build your emotional regulation, relationship, and mindfulness skills with our expert online DBT therapy videos.
Our DBT therapists provide tips and guidance to help you integrate core DBT techniques into daily life. Whether you want to better manage BPD, emotional dysregulation or just improve your overall mental wellbeing, our YouTube channel offers easily accessible support for your DBT journey.
Subscribe today to start mastering DBT skills and transforming your mental health with DBT London’s online DBT therapy resources.
Get Personalised DBT Therapy in London from Experts
At DBT London, our team of accredited experts has over a decade of experience in providing specialised DBT treatment in London and through online and in-person platforms. We’re deeply committed to fostering your journey towards emotional resilience, stability, and enhanced mental wellbeing.
Experience transformative DBT therapy in London with our compassionate therapists. Whether you’re struggling with borderline personality disorder (BPD), emotional dysregulation, self-harm tendencies, or relationship challenges, we’re here to offer you personalised care. Our approach is tailored to meet your unique needs and overcome specific challenges.
We take pride in guiding our clients at DBT London towards a path of positive transformation. Our goal is to help you lead a more balanced and fulfilling life. We address a diverse range of emotional challenges with our bespoke in-person and online DBT therapy sessions.
Embark on your path to improved mental health with our experienced DBT therapists in London. We are dedicated to supporting you through your personal challenges, helping you develop crucial emotional regulation skills and resilience.
Contact DBT London today to schedule your free consultation. Start your journey with personalized DBT treatment in London that delivers real, tangible results.
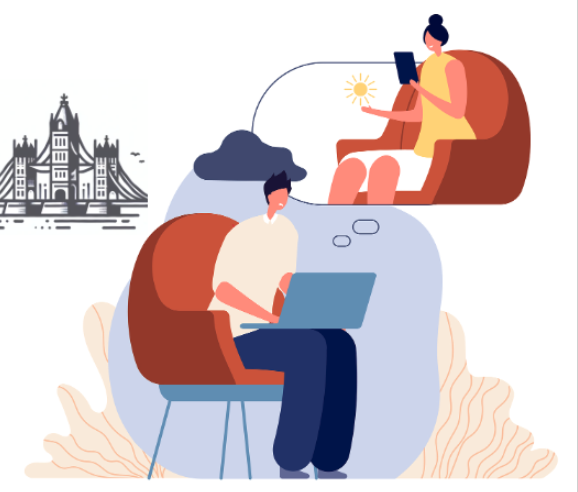
Same Day DBT Assessments